Use Cases
The primary goal of the Streamdal is to make it easy to build and maintain complex distributed systems that utilize asynchronous processes. Because of this, Streamdal is the perfect solution for folks who are actively utilizing or migrating to event driven architectures such as event sourcing.
However, due to the nature of the Streamdal platform, our product can also be used piecemeal to facilitate non-event-driven use-cases as well such as smart CDC, global dead-letter queue, disaster recovery and much more.
Below are some of the many ways companies are utilizing Streamdal.
Platform Rearchitecting
Problem: Your organization has decided to utilize an event driven architecture, but there are so many things that need to be custom built.
There are some core functionalities you should absolutely consider having for event-driven. You need a process for:
- Storing messages (indefinitely)
- Searching all messages
- Viewing searched messages (especially if messages are encoded)
- Replaying messages
Every one of these things is a massive undertaking and will likely take months to complete, and will require ongoing maintenance.
Solution: Our platform addresses all of the above problems and more. Streamdal is a fully managed platform that provides a robust set of features that can be used to build and maintain any event driven system.
Our platform enables you to:
- Ingest any number of messages/events
- Supports virtually all messaging and streaming systems (see full list here)
- Supports all major encoding formats (see full list here)
- Supports granular, full-text search across ALL events
- Supports granular replay of data into any destination (such as Kafka, Kinesis or GCP Pub/Sub; see full list here)
- Includes robust schema management, validation and conflict resolution features
Messaging System Observability
Problem: Messaging systems are a black box, and you need a way to see what is flowing through your bus or message queue.
This is an extremely common use case, and one of the primary reasons we built Streamdal. It should be trivial for anyone to see whatâs inside of your message queue - without having to ask devops or your data team to give you a dump of messages.
For example:
- You are a backend developer tasked with implementing a new feature in a
billing
service that you have never touched before - You know that
billing
consumes and produces messages to Kafka, but you donât know what the messages look like or what theyâre supposed to contain - Prior to starting development, it would be nice to âseeâ actual messages (without having to look through other codebases to see how a message is constructed or how it is read)
Solution: Streamdal gives observability of all data flowing through your messaging systems regardless of encoding. You will be able to view all data in a human-readable format. Read more about our supported encodings here.
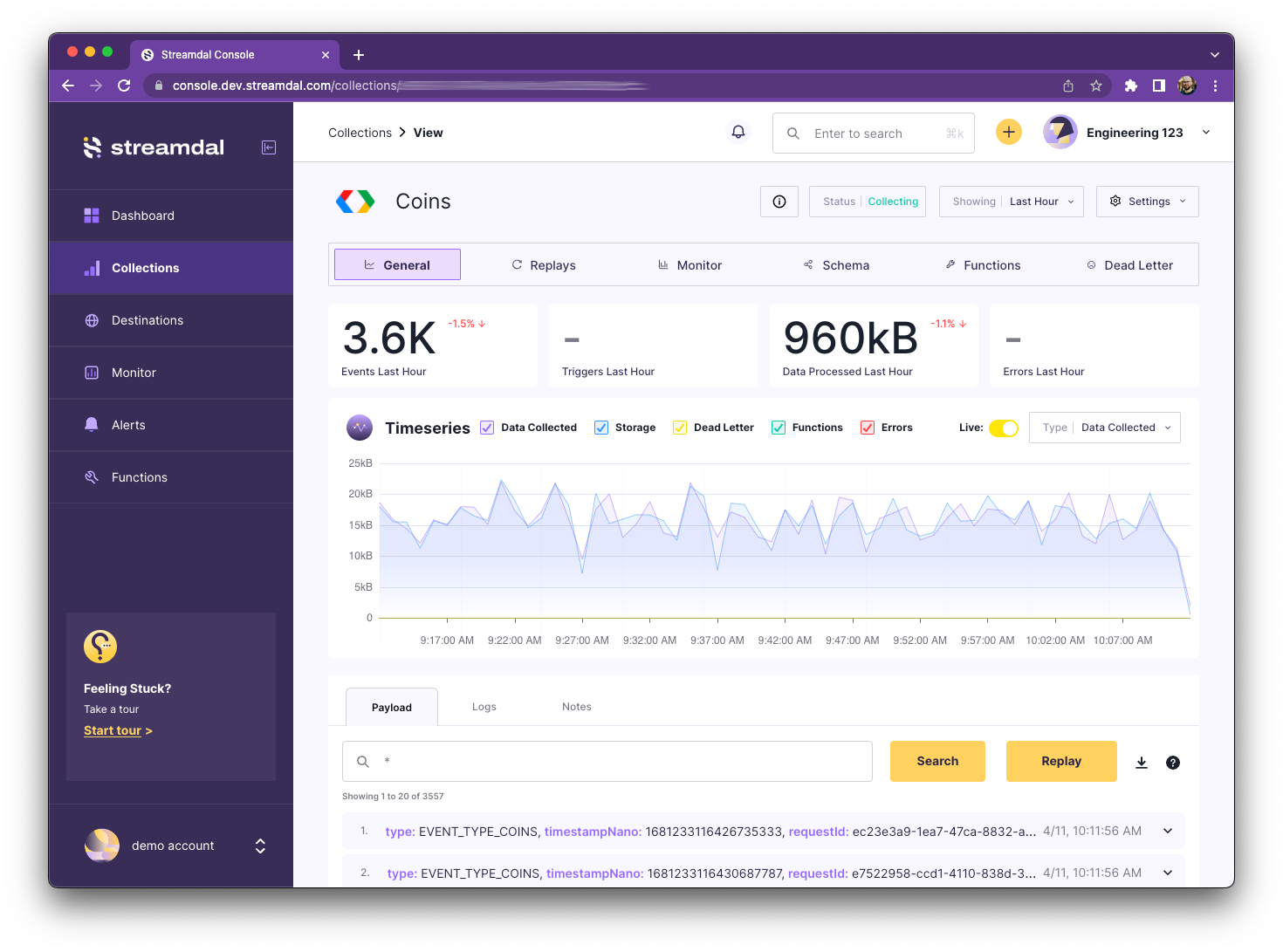
Wth Streamdal it takes just two steps to start viewing data in your streams, and we provide other massive observability benefits. Check out our quickstart guide here.
Data Science
Problem: Data needs to be cleaned up (such removing bad characters, garbage text, etc), parsed, and mutated into a useable form such as JSON or Parquet.
On top of that, there are many different data sources that data science teams can use - traffic logs, application logs, 3rd party data (ie. Salesforce, Segment), databases and many more, but often, the most useful is the data on your message bus that your backend systems rely on to make decisions.
For example, your order processing, account setup or email campaign process all might use a message bus such as RabbitMQ or Kafka to asynchronously achieve the tasks at hand.
Gaining access to such a rich data source would be huge, but it will likely take quite an effort because:
- The data science team would need to talk to the folks responsible for the message bus instance (say, RabbitMQ in this scenario) to gain access
- Everyone involved would need to come to a consensus on what data the data science team needs, and periodically reevalute as data and business needs grow
- Someone (usually the devs or devops) would need to figure out how to pull the associated data and where to store it for data science consumption
- As a best case scenario, someone might implement a robust pipeline with an off-the-shelf solution (that wonât break in 3 months), but more often this will consist of writing a script or two that is ran on an interval and periodically dumps data to some (to be determined) location
And finally, the data science team has access to the data! đ«
Solution: All data ingested into Streamdal is automatically indexed into a data science friendly parquet format, and with our Replay functionality, you can simplify the labyrinth of ETL pipelines.
All you have to do is:
- Hookup plumber to your message bus
- Have your data science team log into the Streamdal dashboard and query their desired data
- When good candidate data is found, the data science team can initiate a replay that includes ONLY the events they are interested in, and point the destination to their dedicated Kafka instance (or whatever tech theyâre using)
- The replayed data will contain the original event that was sent to the event bus
In the above example, we have saved a ton of time - the data science team can view AND pull the data without having to involve another party, and without having to write a line of code.
Smart Dead Letter Queue
Problem: Data anomalies periodically arise causing complications and downstream consumption issues. Problematic events/messages are then published to a Deadletter Queue (DLQ), but resolving these incidents often involves a lot of tools, code, and time.
Some messaging systems like Kafka natively support Deadletter topics/queues, but after the incompatible messages get sent to the DLQ, the heavy lifting is left up to engineering for resolving these issues such as:
- Finding out what parts of the messages/events are incompatible with your schema
- Writing/modifying scripts to read, fix, and replay data back into your system for reprocessing
If you are using encoded data, the lack of observability will significantly prolong and exacerbate the recovery process.
Solution: With Streamdal, all DLQ data will visible, searchable, and easily repairable (regardless of encoding) with our first of itâs kind Smart Deadletter Queue (DLQ).
With our Smart Deadletter Queue, you can:
- Fully observe all data with schema incompatibilities or payload anomalies
- Repair data manually for small fixes, or enmasse with serverless WASM functions
- Replay fixed data back into your system for reprocessing, or to any destination
In addition to these capabilities, you can also write data directly to our Smart Deadletter. Read more about Smart Deadletter Queue here
Disaster recovery
Problem: You are tasked with developing disaster recovery mechanisms for production.
Implementing a disaster recovery mechanism is not easy⊠or fun. It is usually (and unfortunately) an afterthought brought on by a new requirement in order to get certifications or land a client.
And while there is no argument that having a disaster recovery strategy is a good idea - it does require a massive time investment that usually involves nearly every part of engineering. But it doesnât have to be that way.
Full disclosure: This use-case assumes that your company is already utilizing event sourcing - your events are your source of truth, and thus your databases and whatever other data storage can be rebuilt from events.
If that is not the case, Streamdal will only be a part of your disaster recovery strategy and not the complete solution.
Why DIY is NOT the way to go
Most/common disaster recovery mechanisms:
- Represent the CURRENT state of the system - they do not evolve
- Are brittle - if any part of the stack changes, the recovery process breaks
- Are rube goldberg machines
- Are built by engineers already on tight deadlines - corners will be cut
- Are not regularly tested
The end result is that youâve built something that wonât stand the test of time and will likely fail when you need it the most.
Solution: With the combination of event sourcing and Streamdal, it is possible to create a solid and reliable disaster recovery strategy that does not involve a huge work force or periodic updates.
Assuming that your message bus contains ALL of your events, you can hook up Streamdal to your messaging tech and have us automatically archive ALL of your events and store them in parquet, in S3. Read more about data ingestion here
If/when disaster strikes (or performing periodic disaster recovery test runs), all you have to do to ârestore stateâ is to replay ALL of your events back into your system.
An added bonus: If a 3rd party vendor (such as Streamdal) is unavailable - you still have access to all of your data in parquet. You can implement a âpanicâ replay mechanism that just reads all of your events from S3, bypassing the need to have Streamdal perform the replay.
Our experience with disaster recovery
We have participated in multiple disaster recovery initiatives throughout our careers. In almost all cases, the implementation process was either chaotic, short staffed, underfunded, or all of the above.
While building Streamdal, we realized that this is exactly the type of solution we wished we had when we previously worked on implementing disaster recoviery strategies. It wouldâve saved everyone involved a lot of time, money, and sanity.
If you are working on implementing a disaster recovery mechanism utilizing event sourcing - talk to us. Weâd love to hear your plans and see if we can help.
Continuous Integration Testing
Problem: You are building a new service/feature, and running tests using fake or synthetic data inconsistently surfaces functional errors.
Here is a common example:
- Your software consumes events from your message queue and consumes data within the message to perform XYZ actions
- Your tests have âfakeâ or synthetic event data - maybe your local tests seed your message queue, or maybe you just inject the fake data for tests
Injecting fake data is OK, but it is most definitely not foolproof. If your event schema has changed OR if your data contents have changed - maybe âtimestampâ used to contain a unix timestamp in seconds but now contains unix timestamps in milliseconds - How do you ensure consistent and effective testing?
Normally, youâd find out about this at the most inopportune time possible - once the code is deployed to dev⊠or worse, into prod. đŹ
Solution: With Streamdal, you can avoid the above scenario entirely by instrumenting replays to send chinks of the latest, specific event data directly from your dev message queues as part of your CI process.
This way, rather than using synthetic data in your tests, you would use real event data that is used in dev and production. The result is more confidence in your software and in your tests.
Load testing
Load testing is a hot topic - there are MANY ways to do load testing and there are equally as many tools to make it happen. Most load testing strategies involve more than one tool, and more than one logical process.
Load testing tooling falls into two typical categories:
-
Performance oriented
- Verify that youâre able to handle N/req per second
>10,000 req/s
- Verify that youâre able to handle N/req per second
-
Scenario Oriented
- The ability to chain many requests together in a specific order at
ârelatively highâ speeds
<10,000 req/s
- The ability to chain many requests together in a specific order at
ârelatively highâ speeds
Streamdal would fall into the performance oriented category.
Solution: While most load testing tooling is geared towards HTTP testing, Streamdal is unique that you are able to load test your service message consumption functionality.
In other words - as part of your CI process (or another external process), you can test how well your service is able to consume a specific amount of messages that arrive on your message bus.
The process for implementing this would be fairly straight forward:
- Connect plumber with your message queue
- Using the API or the dashboard, create a destination (A) of where events will be replayed to
- Using our API, create a replay using a search query with the destination set to (A)
- Start the replay
- Instrument and monitor how well and fast your service is able to consume the replayed events
Data Lake Hydration
Problem: Your data ops for business/analytical needs require efficient storage and retrieval for both structured and unstructured data.
Before we dive into this - letâs establish what is a âdata lakeâ:
A data lake is a centralized repository that allows you to store all your structured and unstructured data at any scale.
..
A data warehouse is a database optimized to analyze relational data coming from transactional systems and line of business applications.
..
A data lake is different, because it stores relational data from line of business applications, and non-relational data from mobile apps, IoT devices, and social media.
Source: https://aws.amazon.com/big-data/datalakes-and-analytics/what-is-a-data-lake/
To simplify it further - your data lake may contain huge amounts of âunorganizedâ data without any advance knowledge as to how youâll be querying the data. Whereas a data warehouse will likely be smaller and already be âorganizedâ into a usable data set.
All of this roughly translates to: if you make business decisions from your data, you will likely need both. If you donât have a data lake, you may be wondering: How do you create a data lake?
This is where Streamdal comes in beautifully.
Solution: Because we are storing your messages/events in S3 in parquet (with optimal partitioning), we are essentially creating and hydrating a functional data lake for you.
When Streamdal collects a message from your message bus, the following things happen behind the scenes:
- We decode and index the event
- We generate (or update) an internal âschemaâ for the event
- We generate (or update) a parquet schema
- We generate (or update) an Athena schema and create/update the table
- We store the event in our hot storage
- We collect and store the event in parquet format in S3 (using the inferred schema)
You own this data lake and can use the events however you like, whether by using Streamdal replays, Athena searches, or piping the data to another destination.
Backups
Problem: You need an efficient way to back up the data in your message queues.
More of often than not, message queues contain important information that are critical to operating your business. While some tech like Kafka retains messages, most messaging tech has no such functionality.
The common approach to this is to write some scripts that periodically backup your queues and hopefully, if disaster strikes, your engineers can cobble the backups together.
Solution: With Streamdal, there is no need to perform periodic backups. Messages are âbacked upâ the moment they are relayed to Streamdal and are immediately visible and queryable.
Streamdal replays enable you to restore your message queue data without having
to write a single line of code. Just search for *
and replay the data to your
message queue. This is also somewhat related to how you can use Streamdal for
disaster recovery.